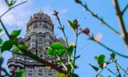
Workshop on Surface Dynamics on the occasion of Patrice Le Calvez's 60th anniversary. Scheduled to take place from December 17 to 20, 2018 in Montevideo, Uruguay.
Workshop on Surface Dynamics on the occasion of Patrice Le Calvez's 60th anniversary. Scheduled to take place from December 17 to 20, 2018 in Montevideo, Uruguay.
The second IMAGINARY conference on open and collaborative communication of mathematical research will be held from December 5 to 8, 2018 in Montevideo. We invite you to join this interactive conference which offers talks, workshops and a great cultural program!
Colaboración en Matemática y Equidad de Género en el Liderazgo: Matemáticas en el Cono Sur es un taller de colaboración entre investigadoras e investigadores sobre problemas de matemática que actualmente no están resueltos. El foco de este taller es trabajar en conjunto para avanzar en problemas de actualidad y no en la exposición de resultados como es habitual
Los Encuentros Rioplatenses comenzaron a realizarse hacia fines de 1996, alternativamente en Montevideo y Buenos Aires. En 2016 se realizó por primera vez en la ciudad de La Plata. El objetivo principal de esta actividad es fomentar el encuentro tanto entre investigadores de la región como entre estudiantes avanzados de grado y posgrado.
Workshop on Groups, Geometry and Dynamics. A satellite event of the International Congress of Mathematicians 2018. With a homage to Ricardo Mañé.
ICT4V y el Centro de Análisis de Big Data (CABIDA) se complacen en anunciar el primer workshop “Big and Complex Data Theory, Applications and Value Creation”
En 2017 continúa la exhibición IMAGINARY recorriendo capitales del país. Las próximas ciudades...